Diffusion Models
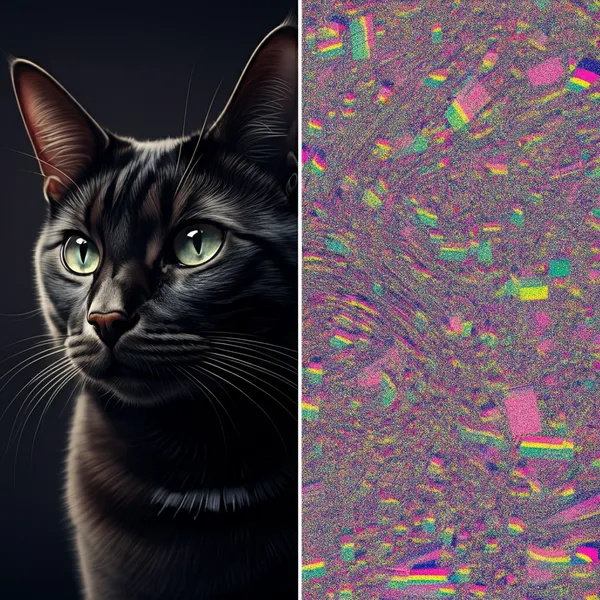
Three speakers giving three distinct talks on Diffusion Models.
Evelyn J. Boettcher will give a high level talk on what diffusion models .
PhD student Daniel Brignac will do a deep dive on the math, followed up with
Wesley Giles giving a MLOPs example of how diffusion models are used.
What are Diffusion models.
Diffusion models are a type of generative AI model used to create new data, such as images or audio, from random noise:
- Starting point: Begin with random noise.
- Forward process: Gradually add noise to real data samples until they become indistinguishable from random noise.
- Reverse process: Train a neural network to learn how to remove noise step-by-step.
- Generation: To create new data, start with random noise and use the trained model to progressively remove noise, revealing a new, coherent sample.
Key points:
- Diffusion models learn to denoise data
- They work iteratively, making small improvements each step
- The process mimics how information spreads or "diffuses" in nature
- They've shown impressive results in image and audio generation
The Gem City Machine Learning (ML) group is part of the GemCity TECH family of user communities in Dayton OH.